The Four Stages of Data Modernization for Digital Transformation
Digital Transformation (DX) is crucial to every business, from small-scale enterprises to medium-sized businesses to multinational corporations. Our world is becoming increasingly digital, and how a company carries out its DX initiatives determines its competitiveness and relevance today.
Since the term ‘digital transformation’ means different things to different businesses, it is hard to define it. However, in general terms, digital transformation is the integration of digital technology into every key area of a business that impacts the fundamentals of its operations and its value delivery to customers.
Beyond that, DX is a cultural reform in which enterprises continually challenge the status quo and get comfortable with trials and errors. This also means that the business organizations should walk away from legacy business operations on which the company was built and embrace new business practices.
What Is Data Modernization?
In today’s digital Information Age, unimaginable volumes of data get generated every second from countless devices and sources in structured and unstructured formats. The mixture of structured data (such as documents and spreadsheets) and unstructured data (blog posts, videos, and social media comments) brings storage and data processing challenges.
Unfortunately, most organizations struggle to put this enormous data into effective use without realizing two essential things. First, their legacy data architectures stand in the way of generating deep insights for effective decision-making. Second, data modernization is the key to unlocking the limitless potential of data processing.
Data modernization helps enterprises move siloed data from legacy databases to modernized cloud-based databases or data lakes. Legacy systems have several inefficiencies, complexities, and bottlenecks. An enterprise that embraces data modernization eliminates those obstacles and turns into an agile one. So, data modernization is the foundation of digital transformation in an absolute sense.
The Four Stages of Data Modernization
Let’s explore the four stages of an efficient data modernization process toward successful digital transformation.
- Data Migration
Data migration is the first step of data modernization and most DX projects. Unfortunately, in most cases, the professionals complicate the process or tend to prematurely transform the data before data migration. Instead, they could have performed a lift and shift migration: a process that allows quicker data migrations and lets organizations leave legacy systems faster.
- Modernization of Data and Application
After the data has been migrated to the cloud, the data and application modernization phase commences. As it is carried out on the cloud, this phase enables a wide range of capabilities that are difficult to attain from on-premises (on-prem) systems. Some examples are real-time collaboration, easily accessible data sharing, and a more straightforward yet more informative Business Intelligence (BI) dashboard.
- Implementation of Modern Analytics
Data modernization empowers an enterprise to obtain more meaningful insights from data. And with modern analytics, an organization can learn more about their customers, identify customer behavior patterns, and make more informed decisions.
In addition, connecting multiple data sources to cloud-based modern analytics is simpler than connecting on-prem databases to similar data sources. Cloud-based data pipelines are easier to build and smoothly navigate through problems such as data gravity that on-prem databases fail to deal with.
- Apply Artificial Intelligence and Machine Learning for Innovation
Businesses have been using Artificial Intelligence (AI) and Machine Learning (ML) to overcome several business challenges in recent years. The last stage of data modernization is to unlock the innovation potential of AI and ML.
Some AI/ML use cases include manufacturing companies using AI/ML solutions to reduce waste by AI-based predictive maintenance. In addition, AI/ML solutions have been used by organizations to create customer profiles, learn more about customer behavior patterns and devise marketing strategies based on these profiles and forecasts.
Sailing Smoothly Through Data Modernization
According to a study conducted by Statista, 34% of respondents confirmed that their organization had fully implemented data modernization technology, while 50% stated that their organization is currently undergoing the implementation process.
Data modernization may still be a daunting, time-consuming process for some, even after breaking it down into four stages. It is a fact that if conducted in a standalone manner, the data modernization process wastes tons of your time and resources. The lack of continuity is one of the crucial challenges most enterprises face when implementing data modernization.
The best way to overcome this challenge is to shake hands with an Information Technology (IT) consulting firm like GoDgtl. With a focus on helping clients transform their off-line organizational activities and legacy business processes, we can help you modernize your data stack and enjoy modern and rich data experiences.
Recent Posts
In the current business world, data is the most valuable asset, and a disaster involving data loss results in several irreversible damages to an enterprise. Those damages include the loss of revenue, productivity, reputation, and even loyal customers. Disasters and their severity are hard to predict. Anyway, you can control how you respond to a disaster, and your response determines how successful your enterprise will recover from it.
The adoption of cloud computing services has been rising since the COVID-19 pandemic. A recent survey revealed that 67% of enterprises adopted cloud infrastructure by the end of 2021. Cloud computing services deliver on-demand Information Technology (IT) services from storage to applications to processing power via the internet on a pay-as-you-go basis. An enterprise can access all those IT assets by paying a fee rather than owning IT infrastructure or data centers.
Here, let’s take a look at Cloud Disaster Recovery (CDR) and how you can use it to your advantage.
Cloud Disaster Recovery: What is It?
CDR is a cloud-based service that quickly recovers your enterprise’s critical systems after a disaster and gives you remote access to your IT resources in a highly secure virtual ecosystem.
Conventional disaster recovery involves managing a secondary data center, a time-consuming yet expensive process. However, cloud disaster recovery has transformed the status quo by eliminating the need for traditional IT infrastructure and bringing down downtime significantly. According to the 2021 Data Protection Report by Veeam, the average downtime cost is roughly $85,000 per hour. And, the cost entirely depends on the size of the business organization – the larger the size, the larger the cost, and vice versa.
The Working of Cloud Disaster Recovery Explained
To understand the working of cloud disaster recovery, we should compare it with conventional disaster recovery. As mentioned earlier, the crucial element of conventional disaster recovery is a secondary data center where you can store all redundant copies of critical data and to which you can fail over workloads.
A conventional on-premises disaster recovery system generally includes the elements mentioned below.
- A dedicated ecosystem facilitates IT infrastructure, including maintenance, employees, and computing devices.
- Adequate server capacity for higher levels of operational performance that also allow scalability depending on the business needs.
- Internet connectivity with ample bandwidth allows remote access to the secondary data centers.
- IT network infrastructure, including firewalls, routers, and switches, are implemented to provide data availability and a reliable link between the primary and secondary data centers.
Conventional disaster recovery is often too complex to manage and monitor. More than that, maintenance and support of a physical on-premises DR site can be costly and time-consuming. For example, the expansion of the server capacity of an on-premises data center can only be done by purchasing additional computing devices and IT resources, which demands a lot of money, time, and effort.
The Advantages of Cloud Disaster Recovery
Cloud disaster recovery can effectively deal with most issues of conventional disaster recovery. Some of the advantages of CDR are mentioned below.
- Eliminates the need for a secondary on-premises physical site and the purchasing of additional hardware and software to carry out critical operations.
- Scalability of IT resources per the business needs
- The affordable pay-as-you-go pricing model requires you to pay only for the cloud computing services you use.
- CDR can be performed in minutes from anywhere in the world over any computing device connected to the internet.
- The backup of data across multiple geographical locations eliminates the possibility of a single point of failure. Even if one cloud-based data center fails, you can still retrieve a backup copy of your critical data.
- Cloud-based state-of-the-art IT network infrastructure ensures that the cloud services provider quickly identifies and rectifies any issues or errors. Moreover, the cloud service provider provides 24/7 support and maintenance of the cloud storage and consistent updating of hardware, software, and cybersecurity features.
Disaster recovery in the cloud is increasingly becoming a popular choice for small and medium-scale businesses looking to implement a robust business continuity strategy. With CDR, setting up a separate data center for backup is no longer required. Moreover, there is no need to install and maintain separate DR tools, which brings down costs while providing businesses access to continuous, scalable DR services.
Recent Posts
May, 2022
The digital revolution in healthcare is faster than ever, making public health all the more inclusive, efficient and sustainable. Healthcare organizations are embracing new, data-centric technologies to handle the constant strain on global healthcare systems due to several factors. Healthcare data amounts to almost 30% of the world’s total data volume, while the compound annual growth rate of healthcare data is projected to reach 36% by 2025. That’s 10% more than financial services, 6% higher than manufacturing and 11% faster than media & entertainment.
Data-driven healthcare and cloud solutions are helping healthcare professionals lower costs, provide better care and improve satisfaction. These technologies make it easier for hospitals to align business outcomes with patient outcomes by stimulating processes as well as operational efficiency. Life Sciences and pharmaceuticals have previously relied on experimental observation and costly clinical trials. Recent developments in data sciences have allowed researchers to streamline complex studies. With increasing bandwidth, networking capabilities, and processing power, healthcare information systems are improving with no end in sight. Read more to find out how data and cloud services have increased the scope of impact that quality healthcare has on our lives.
How Data and Cloud Computing Enhance Healthcare Services
Big Data Analytics
Big data is massive amounts of information generated from different sources, stored and analyzed to derive a simpler, more practical truth from the larger picture. Big data has been previously associated with supercomputers, nuclear physics and defense simulations.
Cloud-based solutions like Microsoft Azure can process datasets that are otherwise too large or complex for traditional database systems. As a result, this derivation serves as meaningful information for researchers, patients, doctors, and administrators. For example, healthcare organizations can leverage big data to predict and prepare for a future health crisis.
Big data allows healthcare professionals to drill down and know more about their patients and the effectiveness of the care they provide. Since doctors can identify and draw early warning signs, it becomes possible for them to treat diseases in the early stages. In addition, hospitals can use underutilized health records by merging data from clinics, other healthcare institutes and public health records.
Cloud Transformation
Organizations with bigger employee populations are turning to cloud healthcare solution providers to enable more affordable yet high-quality healthcare services. Cloud provides them with the flexibility, scalability and security needed to keep up with the exponential medical and digital technology advancements.
Leading healthcare organizations have already initiated value-generating cloud transformations that bring various benefits to practitioners and patients alike. Cloud-based solutions help healthcare professionals deal with the ever-increasing operational and infrastructural costs, government compliances, and security concerns. With remote storage, hospitals can access a network of servers where they can safely store large volumes of data.
Cloud offers the amount of interoperability required for advanced healthcare applications such as collaborative patient care, IoT-based devices and quality virtual treatments. For instance, Google Cloud Platform’s (GCP) healthcare data engine makes data immediately useful by providing an interoperable, longitudinal patient data record.
Pharmaceuticals and Research
Data analytics can ensure that the right patient has the right medicine at the right time. Pharmaceutical and research organizations can utilize data to understand diseases better, their progression and prevention and create safer, targeted treatments with minimal adverse events.
Predictive modeling enabled by large datasets can help determine more efficient medications and development processes. More accurate therapy trials with medicines for specific categories of the population or individuals are possible through advanced statistical analysis and better study designs. Big data applied in biomedical research can help us gain novel insights on a granular scale.
This can help with developments in personalized medication and enable a more individualized approach to healthcare. Leading industries in biomedical sciences have leveraged Amazon Web Services (AWS) cloud platform for almost a decade to do more with their data.
Transform to Digital to Let Data Transform You
Today, digital transformation is not merely adopting a bunch of feature-rich software and getting your data online. There are several digital technologies and cloud-based applications to choose from. However, businesses need to go by a proven methodology and incorporate only the right solutions to reach the highest level of efficiency.
GoDgtl has partnered with the industry’s leading cloud platforms to provide healthcare organizations with the full benefits of the modern data landscape. We can help you evaluate the most suitable cloud platform and set up optimal agile processes along with robust security measures across the enterprise.
Recent Posts
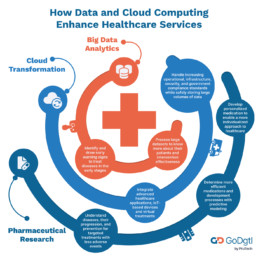
April, 2022
You could be migrating storage information or databases from small to large sizes. In any case, you need to plan ahead in data migration, execute with the right strategy, and ensure data accuracy.
Let us walk through the process in this step-by-step breakdown.
-
Planning
Planning is one of the most critical steps for wild migrating. According to Gartner, “through 2022, more than 50% of data migration initiatives will exceed their budget and timeline—and potentially harm the business.”
Proper planning helps set expected time and success metrics. It clearly lays down the steps with the timeline and expected budget.
- Identify the data and format
- It is possible to migrate in one go in a short timespan, called the Big Bang approach
- Most companies will benefit from migrating in sequences with both systems running, called the trickle approach
- Migration in phases helps avoid downtime
- Make sure enough time has been planned for the migration
- Involve Business units and gather their needs/inputs
- Assess the resources and staffing
- Pick the right tool or build from scratch
-
Preparation and Understanding the Data
Preparation involves auditing the data and creating the backup. It is also the stage when you figure out data governance.
- Refining data helps cut time and cost
- Identify what data you need to migrate
-
Migration Design and Building
Migration design involves freezing upon the approach and detailing the technical architecture of the solution
- Before ETL operations, data needs mapping
- Mapping helps match source fields to target ones
- Cover all documentation by the end of this stage
- Migration design is also where you lay down security plans
-
Execute and Test
This is where you Extract, Transform, and Load the data. According to a survey, 47 percent of the North American IT executives admitted that poor data quality at their company was due to errors during data migration.
- You need to extract the data from the source system and make sure of its integrity
- Once extracted, you transform it to the correct format and load it to the new system
- Testing should begin in the build phase when you start manipulating data
-
Auditing and Maintenance
Once the system is live, you should set up a system to audit the data for accuracy from time to time.
- Use data quality tools to maintain high-quality data
- Maintain with future migrations in mind
Data migration is essential when moving to a cloud-centric environment, which is the current trend. Use data migration to get better performance and competitive advantage.
SOURCES
https://www.bloorresearch.com/technology/data-movement/#emergingtrends
https://www.oracle.com/technetwork/middleware/oedq/successful-data-migration-wp-1555708.pdf
https://www.talend.com/resources/understanding-data-migration-strategies-best-practices/
https://easternpeak.com/blog/7-key-steps-to-perform-a-successful-data-migration/
https://zipreporting.com/en/data-migration/data-migration-process.html
Recent Posts
March, 2022
In the current Information Age, we live through a world of data. Today, everything from an individual to a multinational corporation relies heavily on various types of data and its analytics for their everyday needs. Forbes put forward truly mind-boggling statistics on the amount of data we generate every single day. It estimates that we create, at our current pace, nearly 2.5 quintillion bytes of data each day. With the adoption of the Internet of Things (IoT), this pace is only accelerating.
Despite the ever-increasing volume of information being collected through data analysis today, the functioning of the human mind is the only thing that stays away from change. We think in stories and pictures. Only through the visual representation of data can we interpret it for the benefit of an individual or an organization. Here, the relevance of Data Visualization and Business Intelligence (BI) tools emerges.
What is Data Visualization?
Data visualization is the technique to provide us with a clear idea of the information by giving it visual representation through charts, maps, or graphs. It turns the data into a much more easily digestible format for the human mind to comprehend and quickly identify trends and patterns in larger data sets.
The Benefits of Data Visualization
Data visualization aids an enterprise in quickly recognizing patterns and improves its decision-making process with interactive visual representations of data. Here are some of the benefits of data visualization for a business organization.
Easy Identification of Correlations: Data visualization enables easier identification of correlations between independent variables. Thus, an enterprise can better understand different independent variables and make better business decisions.
Identifying Trends: Identifying trends is the most useful application of data visualization. The necessary data from the past and the present helps an enterprise make futuristic yet accurate business decisions.
Examining Frequency: Data visualization helps to explore the frequency of customers’ purchasing patterns and aids an enterprise in identifying the behavioral patterns of prospective customers towards its different marketing and customer acquisition strategies.
Studying the Market: Data visualization takes the data from different markets, displays it on charts and graphs, and provides an enterprise with insights into which target audiences to focus on and which ones to ignore.
Risk and Reward: Risk and reward metrics should be analyzed through tedious yet complicated spreadsheets and numbers without data visualization. Once the data is visualized, enterprises can quickly home in on areas that may require action.
Swift Reaction to the Market: An enterprise’s ability to obtain visually enriched information easily and quickly on a functional dashboard allows it to proactively respond to ever-changing market conditions and avoid making mistakes.
What are BI tools?
In the modern-day data-driven world, data comes in both structured and unstructured formats from various sources like documents, journals, books, electronic medical records (EMR), images, videos, email, and other business sources.
Business Intelligence (BI) tools are application software that collects and processes large volumes of unstructured data from internal and external sources. They amass data primarily through queries. In addition, these tools prepare data for analysis by helping an enterprise create data visualizations, dashboards, and reports. As per a recent report by Markets and Markets, the global BI market is expected to grow from $23.1 billion in 2020 to over $33 billion by 2025, at a Compound Annual Growth Rate (CAGR) of 7.6%.
Enterprises of every shape and size implement BI tools to manage, analyze and visualize business data or information. More than that, they are helpful for businesses to boost revenue streams and stay competitive in the market. Apart from the few benefits of BI tools for companies mentioned previously, there are a few more ways BI tools can add value to any business.
They are:
- Fast and accurate reporting
- Better, more rapid, and accurate decision-making
- Valuable business insights
- Competitive analysis
- Better data quality
- Increased customer satisfaction
- Identification of market trends
- Improved operational efficiency
- Lower margins
Every Business Needs Data Visualization and BI Tools
BI tools help an enterprise to carry out data visualization. So, the implementation of both these technologies in businesses empowers business leaders and employees to speed up and improve their decision-making and operational efficiency.
Enterprises must be power-packed with informed decisions and enhanced performance to stay ahead in today’s rapidly evolving yet highly competitive business world. BI tools and data visualization have all it takes to make this happen. The belief that BI tools and data visualization techniques are meant only for large businesses does not stand anymore. They are for everyone, be it a large or small enterprise.
Recent Posts
February, 2022
In today’s Digital Age, tremendous amounts of data are generated every single second from endless sources in various formats. First, there is structured data such as documents and spreadsheets, and now on the other side, there is unstructured data like emails, blog posts, videos, and even Twitter posts thrown into the vast mix of data. A recent study by Statista reveals that the total volume of data created, captured, and consumed globally reached 64.2 zettabytes in 2020. The study also predicts that by 2025, data creation worldwide is estimated to cross 180 zettabytes.
Every business organization in every shape and size all over the globe is pursuing digital transformation today. The generation of larger volumes of data together with global enterprises’ collective effort to transform themselves into digital businesses brings up storage challenges and the trouble of processing those myriad data types in vast volumes. Both these challenges put most business organizations under tremendous pressure to use a larger volume of data efficiently.
It is often legacy data architectures that stand in the way of nearly limitless opportunities to generate insights for effective business decision-making. Today’s massive surge in the volume, variety, and velocity of data baffles legacy databases, and they are bending under the heavyweight of these data challenges. Sooner or later, they may break. The only digital system that desperately needs modernizing is conventional on-premises data architecture. And the answer is data modernization.
What Is Data Modernization?
Data modernization includes extending yet retaining the value of legacy data assets, reusing the data buried in the layers of legacy databases, transforming them to updated modern data architectures, and correlating legacy data assets with the latest high-velocity data assets. It involves developing a scalable, flexible data stack, including modernizing databases without the limitations of many stages, integrations, and complexities. In other words, data modernization is simply moving data from legacy databases to modern databases. It is highly crucial for an enterprise that primarily deals with unstructured data.
Three General Data Modernization Methods
Every business organization has its strategies and objectives. Therefore, data modernization doesn’t come with a one-size-fits-all size. Anyway, enterprises can implement three general data modernization methods depending on their business strategies and objectives.
Data Migration
This method involves moving data to a different data management vendor while the source and target schemas remain the same. Data migration includes migrating code, procedures, etc., which usually brings no significant changes to the application. In addition, an enterprise can utilize automation tools for complete data migration. For instance, moving the data from Sybase, a database management system (DBMS) vendor, to save licensing costs comes under data migration.
Data Conversion
The source and target schemas are different during this data modernization process. Data conversion involves transformations during migration. It is a typical data modernization method during re-engineering an application and modernizing a legacy application. Despite the availability of Extract Transform and Load (ETL) tools, the process is manual. For example, data movement from legacy databases like Indexed Sequential Access Method (ISAM) to Relational Database Management Systems (RDBMS) comes under data conversion.
Database Upgrade
This data modernization method involves upgrading to a newer version of the database management system, which requires no transformation. The deprecated code is replaced, and an enterprise can use automation tools to complete the upgrade. For instance, upgrading from SQL Server 2005 to SQL Server 2012 can be considered a data upgrade method.
The Benefits of Data Modernization
Here are some of the enterprise benefits of data modernization:
- It brings scalability to an enterprise to meet growing data analytics needs
- Efficient integration of new data sources to utilize data at any scale focusing on rising data volumes from multiple data sources
- Reduces the time to derive business insights. That means it provides the ability to find value in data even from streaming real-time data quickly.
- Democratize the process of data access for every business function
- Data modernization offers substantial cost benefits over traditional data management technologies.
Data modernization enables Information Technology (IT) enterprises and business leaders to attain and interpret data to anticipate market trends and improve business outcomes. As a result, a business acquires a solid competitive advantage by deriving actionable insights from enterprise data and data modernization strategies. It also allows organizations to deliver ideal Application Programming Interface (API)-driven application integrations and prompt decision-making in a dynamic business ecosystem.
Recent Posts
February, 2022
The adoption of IoT (Internet of Things) has rapidly changed today’s business world. IoT predominantly helps to capture vast volumes of data from multiple sources. However, the processes of collecting, processing, and analyzing the tremendous flow of data from countless IoT devices have turned complex.
The data-driven nature of present-day businesses requires investments in innovative technologies that can turn this complex process into a simplified yet easily comprehensible one. This is the point where the convergence of IoT and Artificial Intelligence (AI) or AIoT can play a major role in redefining how businesses and economies work.
While IoT comprises devices interacting over the internet, the Machine Learning (ML) capabilities of AI make IoT devices learn from the data they collect. AI-enabled intelligent IoT devices can simulate smart human (at times, superhuman) behavior and decision-making patterns with little or no human interference.
The Rising Trend of IoT and AI Convergence
As a prominent part of their business processes, several businesses worldwide have already embraced the combination of IoT and AI. A 2019 survey reveals that respondents that heavily use AI in their IoT operations exceeded their value expectations. Similarly, a PwC 2019 IoT survey shows that 97% of the companies that have integrated IoT with other technologies such as AI and Blockchain were able to reap the benefits through this investment.
Start-ups and large enterprises alike prefer AI technology to bring out the full potential of IoT. The major leading providers of IoT platforms like Oracle, Amazon, and Microsoft have begun incorporating AI technology into their IoT applications already.
From robotics, self-driving cars and advanced analytics to Industry 4.0 Digital Twins and smart IoT devices, AI-powered IoT is increasingly becoming important across a range of business areas. As a matter of fact, the next evolutionary step for IoT is to embrace AI and drive intelligent automation and decision-making.
How the Blend of IoT and AI Works
At its heart, IoT is all about machines with sensors implanted. These machines, over internet connectivity, transmit streams of data. All IoT devices inevitably follow five basic steps. They are:
- Create
- Communicate
- Aggregate
- Analyze
- Act
The ‘Act’ step solely depends on the ‘Analysis’ step that immediately precedes it. Therefore, the efficiency of an IoT device is determined by its Analysis step. This is the point where AI technology portrays a significant role. While IoT devices provide a multitude of data, AI offers both creativity and context to make smart actions. Therefore, businesses can make smart decisions when an unlimited stream of data delivered by IoT devices is analyzed by AI technology.
Surprising Benefits of AI-Powered IoT
Combining two disruptive technologies like AI and IoT brings a broader range of benefits for both businesses and consumers alike. Some of the most popular benefits are as follows:
Enhanced Efficiency in Enterprise Operations
ML capabilities of AI can crunch huge volumes of data from IoT devices and detect patterns that can’t be done with simple computing devices. It can also predict the enterprise operation conditions and bring solutions for ideal outcomes. Therefore, redundant and time-consuming business tasks are eliminated.
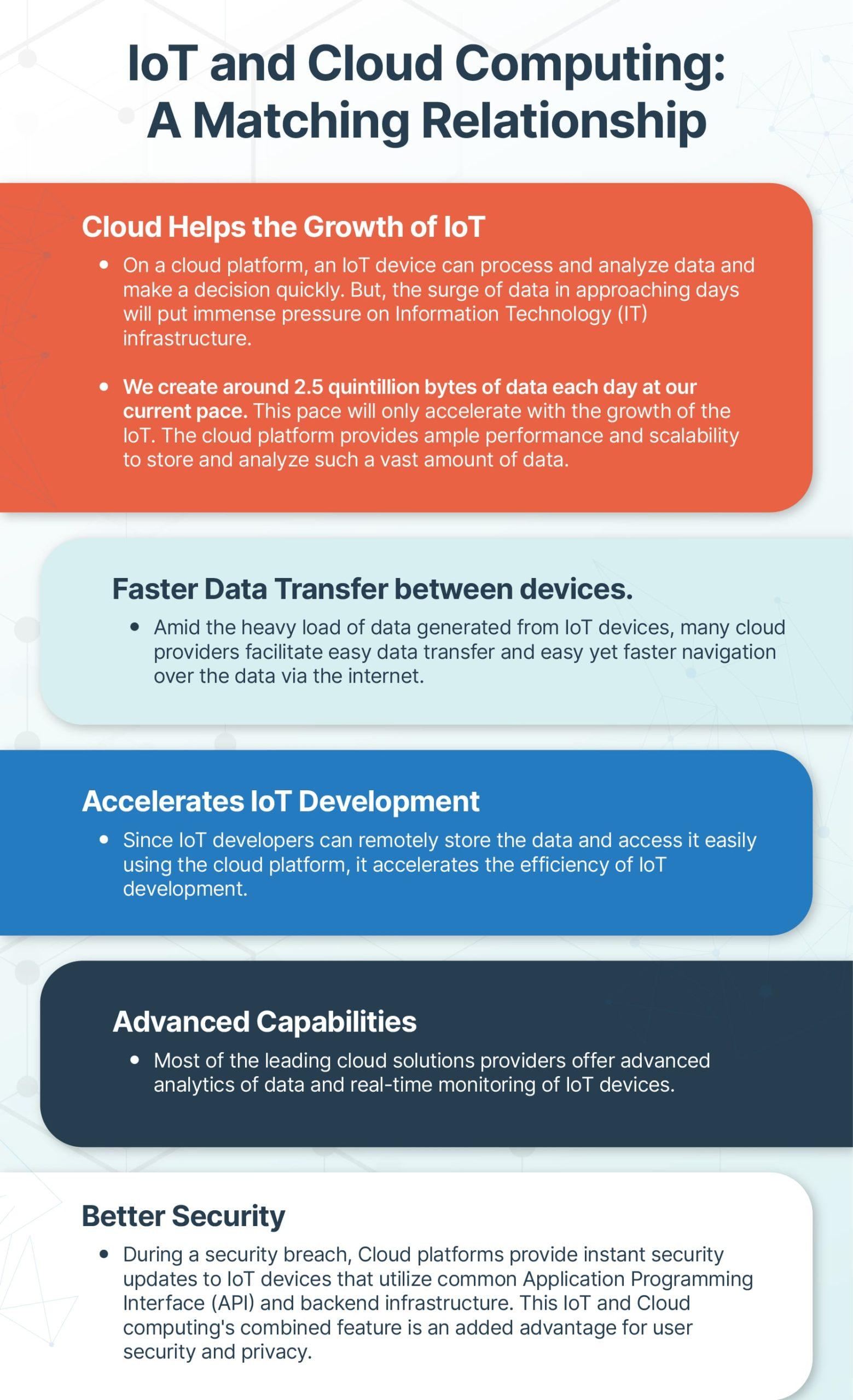
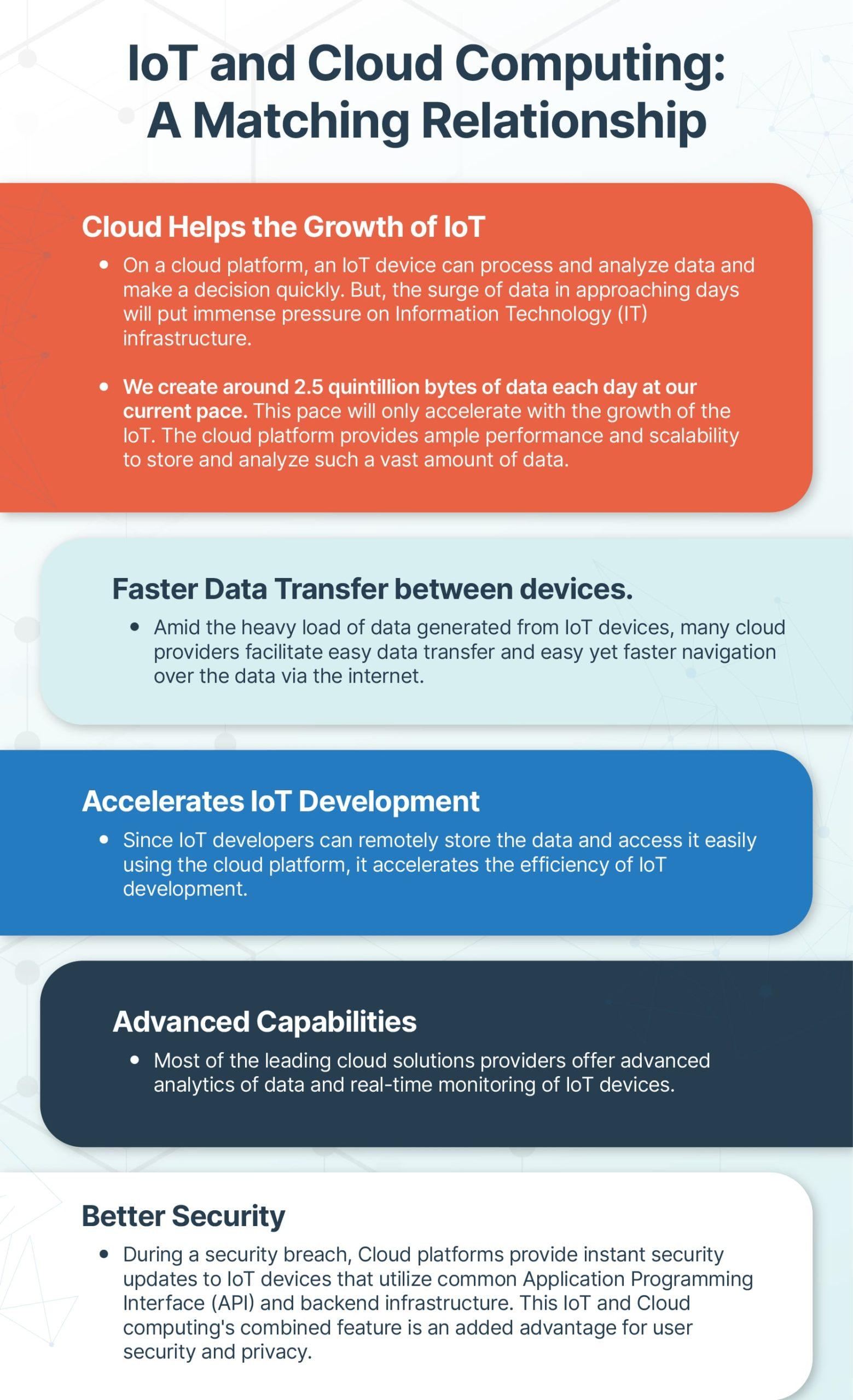
An Efficient Risk Management System
AI-Powered IoT helps businesses analyze and predict a broader set of risks and automate timely responses. It ensures efficient handling of financial loss, employee safety, and cyber-attacks.
Brings Out Innovative Products And Services
A prominent AI technology like Natural Language Processing (NLP) is getting better at letting people communicate easily with computing devices. This leads to the creation of innovative products and services yet enhances the existing ones.
Enhanced Scalability
The AI-powered IoT ecosystem analyzes and crunches the data from devices ranging from smartphones to high-end computing devices to low-end sensors. This process can make large volumes of data handy and make it easier to share across a larger network of IoT devices.
Eliminates Unpredictable Yet Expensive Downtime
In some industrial sectors like offshore oil and gas, as well as industrial manufacturing, unpredictable equipment failures can bring expensive downtime. AI-enabled IoT allows you to predict equipment breakdowns and schedule timely maintenance procedures.
Generally, IoT combined with AI technology can lead the path towards an advanced level of business solutions and user experiences. To achieve greater value from your enterprise network and to transform your business, you should undeniably integrate AI with IoT devices.
Recent Posts
February, 2022
Best Practices for Integrating ML in Your Enterprise
In the present times, Machine Learning (ML) models help solve a wide array of very specific business challenges faced by organizations across industries. Hence, it is important to choose an ML model that aligns with the problem you need to solve. Finally, the approach you choose to build your ML model must be productionable and easily executable for long-term results.
Check out this infographic that highlights the best practices that you can follow when integrating ML models with your business applications.
Best Machine Learning Practices for the Cloud
Cloud or On-Premise?
Choosing the cloud means saving time, easy scaling, and low entry costs. Moreover, there are big players such as Microsoft Azure providing services that allow for easy scaling.
Use Checkpoints
Having checkpoints after every training and evaluation stage using parameters and hyperparameters can help immensely.
Gather Historical Data from the Current Systems
Gathering already existing data can help you understand where optimization is necessary to give you the best results.
Create a Business Objective & Problem Statement
Having an objective or a problem statement helps prioritize. It is a metric that you can optimize and will show you progress.
Use Easily Attributable & Observable Metrics
Using simple metrics, in the beginning, is a great idea. While indirect effects offer great added value in the long run, it is better to start small.
Pass Sanity Checks Before Deploying The Final Model
Testing is the key before you deploy your machine learning model. You can even automate the process and check the generated metrics later to see if it gives you the expected results. Some standard metrics you can deploy are f1 score, accuracy, or recall.
Use Kubernetes & Containers in Deployment
Technologies such as Kubernetes and Docker help you encapsulate different parts of the system. That helps in making incremental improvements, and the scaling process becomes easier.
Takeaway
Deep Learning and Machine Learning have come a long way from being mere buzzwords. Today, they are an integral part of startups and businesses worldwide. Still, many organizations find ML integration to be challenging. However, following these best practices can help them take off in the right direction to eliminate the risks early in the production process.
Sources Used
https://towardsdatascience.com/mlops-practices-for-data-scientists-dbb01be45dd8
https://www.cio.com/article/3623353/best-practices-to-embrace-an-mlops-mindset.html
Recent Posts
February, 2022
The adoption of IoT (Internet of Things) has rapidly changed today’s business world. IoT predominantly helps to capture vast volumes of data from multiple sources. However, the processes of collecting, processing, and analyzing the tremendous flow of data from countless IoT devices have turned complex.
The data-driven nature of present-day businesses requires investments in innovative technologies that can turn this complex process into a simplified yet easily comprehensible one. This is the point where the convergence of IoT and Artificial Intelligence (AI) or AIoT can play a major role in redefining how businesses and economies work.
While IoT comprises devices interacting over the internet, the Machine Learning (ML) capabilities of AI make IoT devices learn from the data they collect. AI-enabled intelligent IoT devices can simulate smart human (at times, superhuman) behavior and decision-making patterns with little or no human interference.
The Rising Trend of IoT and AI Convergence
As a prominent part of their business processes, several businesses worldwide have already embraced the combination of IoT and AI. A 2019 survey reveals that respondents that heavily use AI in their IoT operations exceeded their value expectations. Similarly, a PwC 2019 IoT survey shows that 97% of the companies that have integrated IoT with other technologies such as AI and Blockchain were able to reap the benefits through this investment.
Start-ups and large enterprises alike prefer AI technology to bring out the full potential of IoT. The major leading providers of IoT platforms like Oracle, Amazon, and Microsoft have begun incorporating AI technology into their IoT applications already.
From robotics, self-driving cars and advanced analytics to Industry 4.0 Digital Twins and smart IoT devices, AI-powered IoT is increasingly becoming important across a range of business areas. As a matter of fact, the next evolutionary step for IoT is to embrace AI and drive intelligent automation and decision-making.
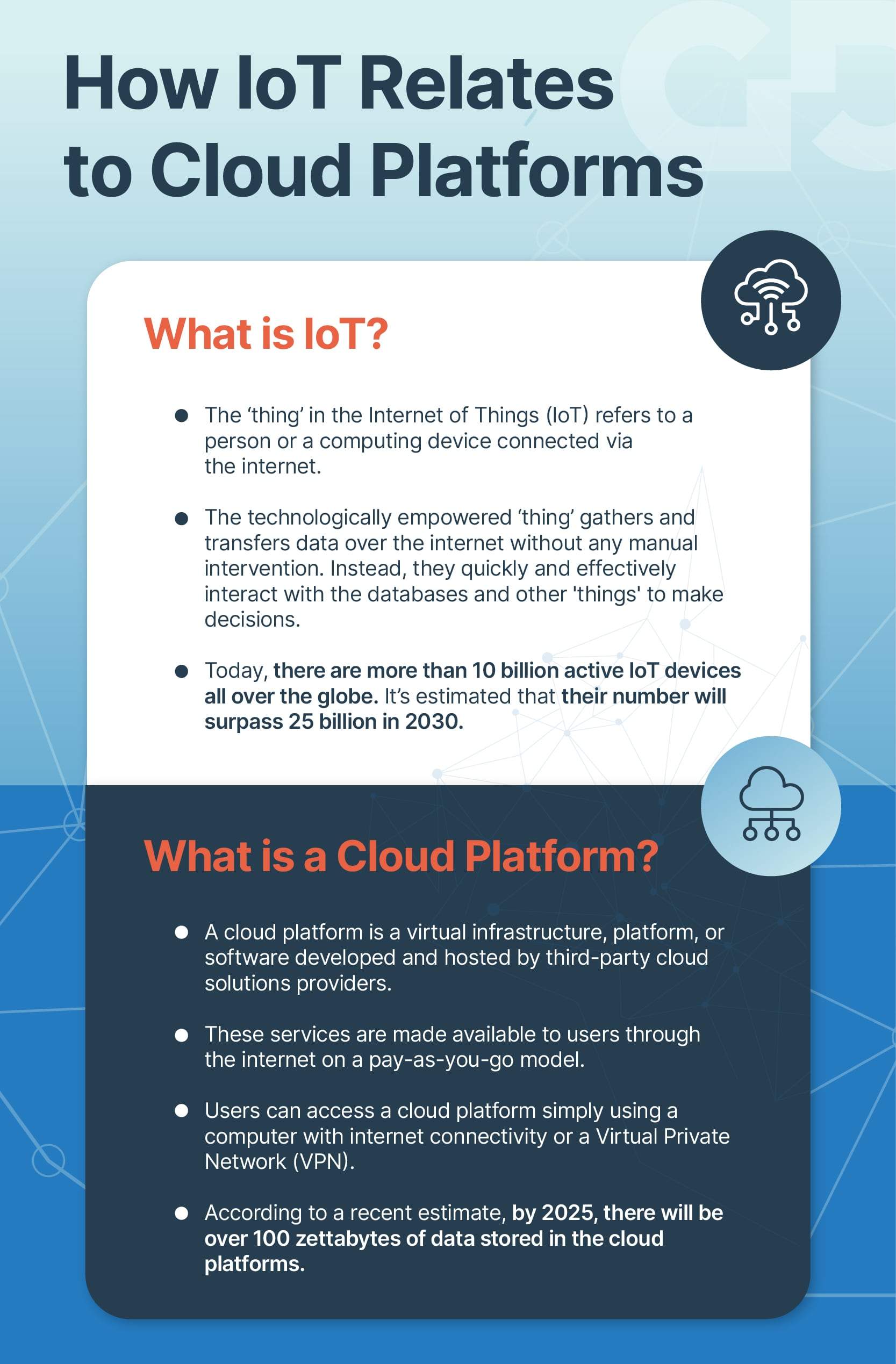
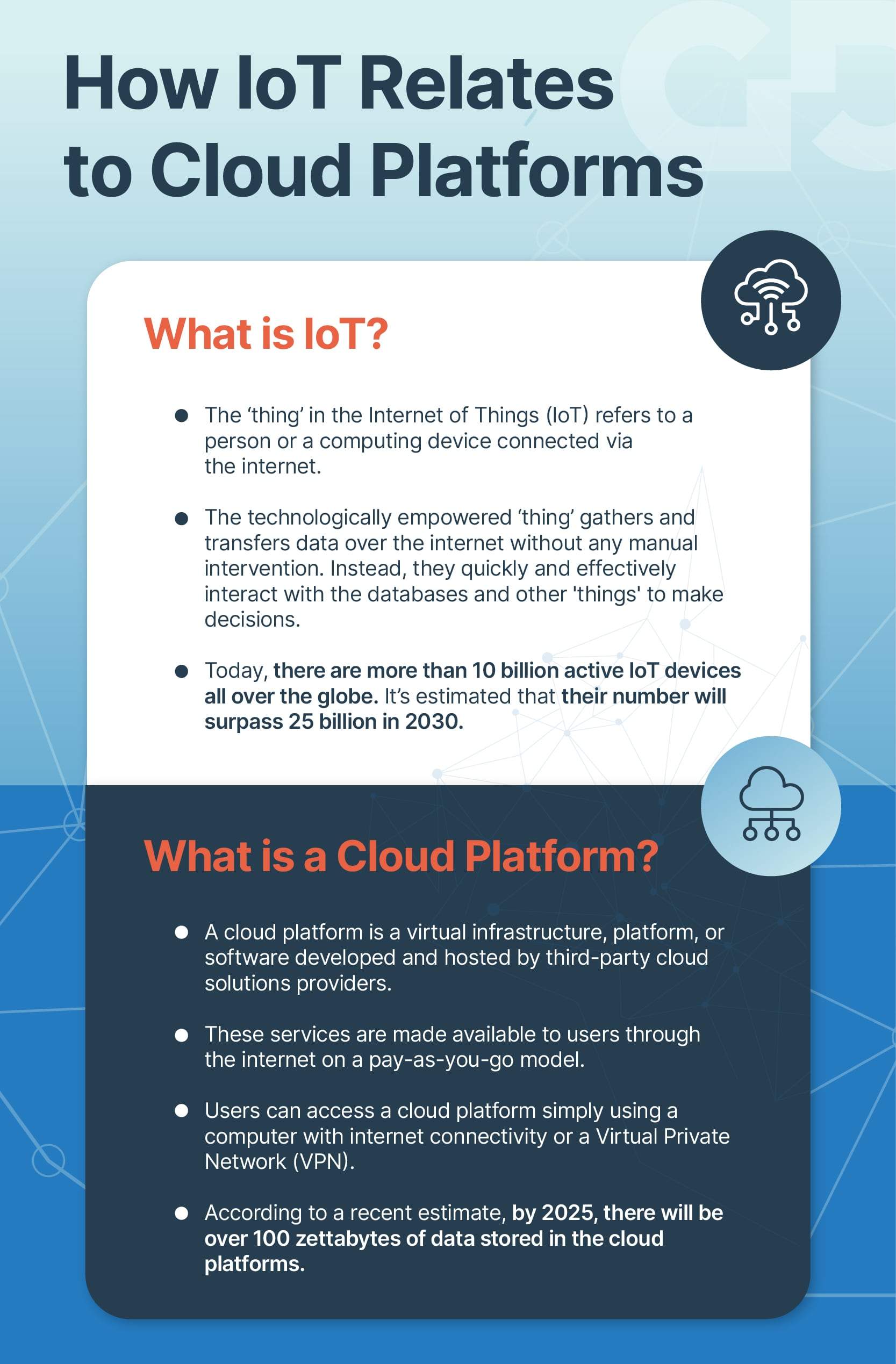
How the Blend of IoT and AI Works
At its heart, IoT is all about machines with sensors implanted. These machines, over internet connectivity, transmit streams of data. All IoT devices inevitably follow five basic steps. They are:
- Create
- Communicate
- Aggregate
- Analyze
- Act
The ‘Act’ step solely depends on the ‘Analysis’ step that immediately precedes it. Therefore, the efficiency of an IoT device is determined by its Analysis step. This is the point where AI technology portrays a significant role. While IoT devices provide a multitude of data, AI offers both creativity and context to make smart actions. Therefore, businesses can make smart decisions when an unlimited stream of data delivered by IoT devices is analyzed by AI technology.
Surprising Benefits of AI-Powered IoT
Combining two disruptive technologies like AI and IoT brings a broader range of benefits for both businesses and consumers alike. Some of the most popular benefits are as follows:
Enhanced Efficiency in Enterprise Operations
ML capabilities of AI can crunch huge volumes of data from IoT devices and detect patterns that can’t be done with simple computing devices. It can also predict the enterprise operation conditions and bring solutions for ideal outcomes. Therefore, redundant and time-consuming business tasks are eliminated.
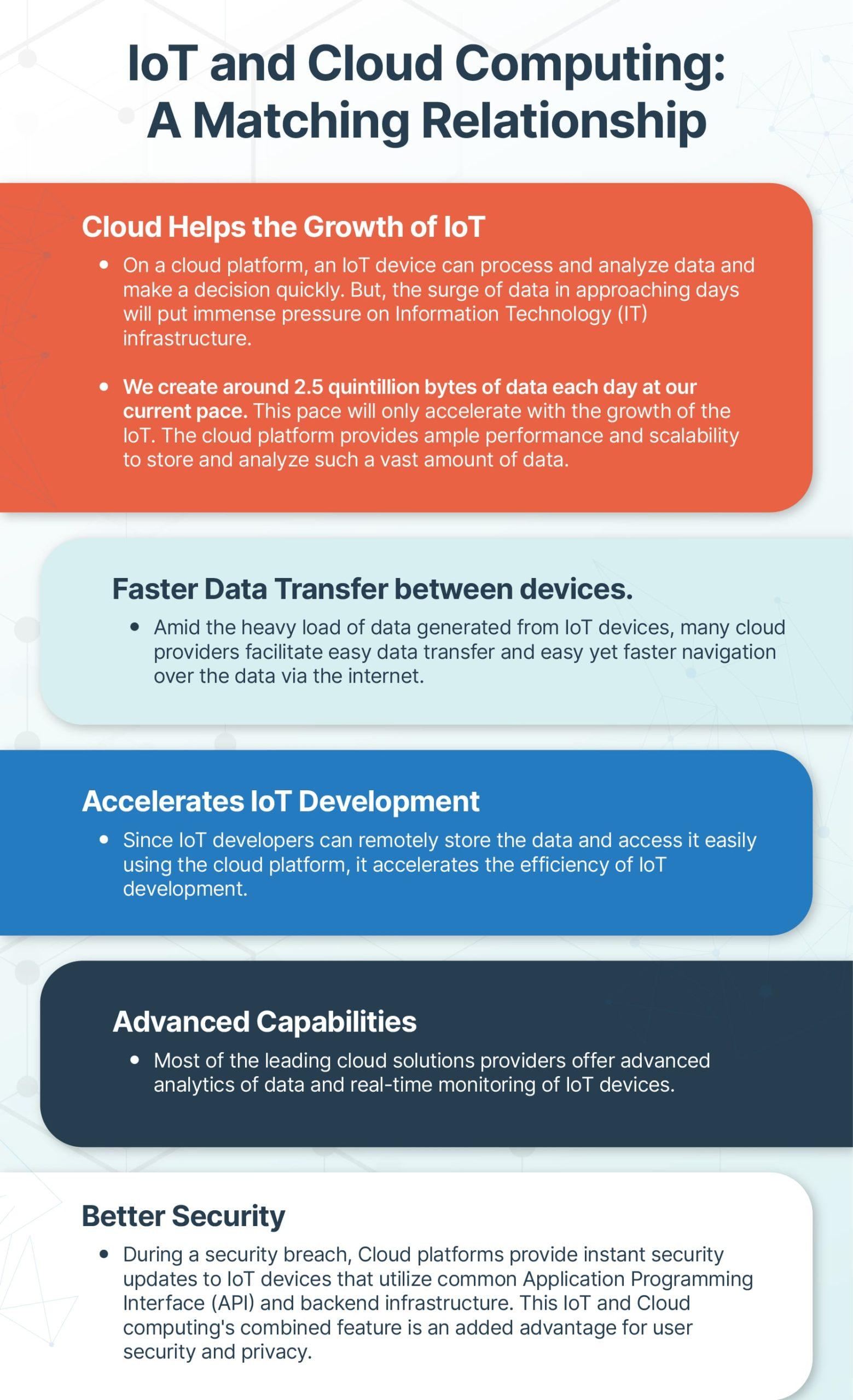
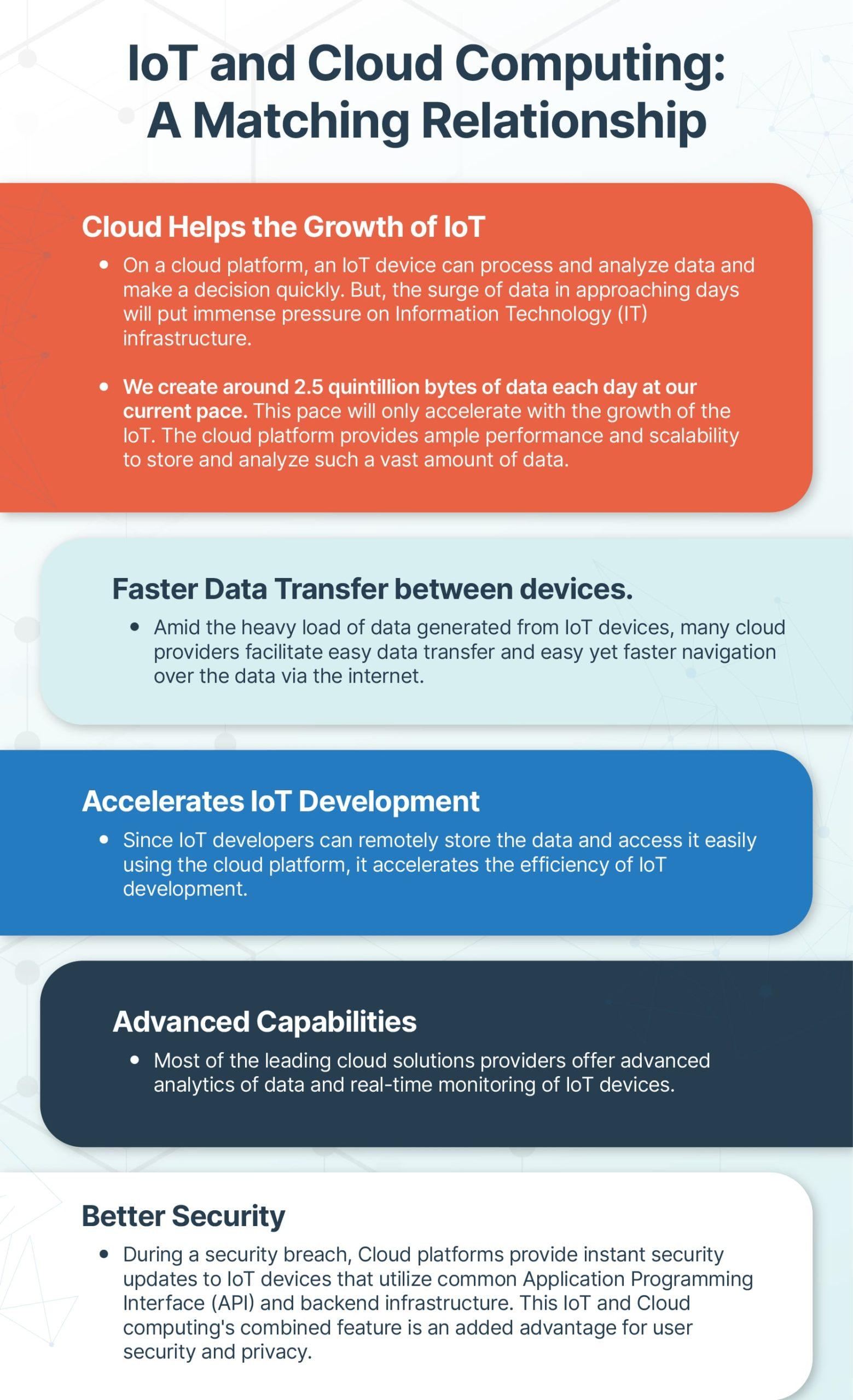
An Efficient Risk Management System
AI-Powered IoT helps businesses analyze and predict a broader set of risks and automate timely responses. It ensures efficient handling of financial loss, employee safety, and cyber-attacks.
Brings Out Innovative Products And Services
A prominent AI technology like Natural Language Processing (NLP) is getting better at letting people communicate easily with computing devices. This leads to the creation of innovative products and services yet enhances the existing ones.
Enhanced Scalability
The AI-powered IoT ecosystem analyzes and crunches the data from devices ranging from smartphones to high-end computing devices to low-end sensors. This process can make large volumes of data handy and make it easier to share across a larger network of IoT devices.
Eliminates Unpredictable Yet Expensive Downtime
In some industrial sectors like offshore oil and gas, as well as industrial manufacturing, unpredictable equipment failures can bring expensive downtime. AI-enabled IoT allows you to predict equipment breakdowns and schedule timely maintenance procedures.
Generally, IoT combined with AI technology can lead the path towards an advanced level of business solutions and user experiences. To achieve greater value from your enterprise network and to transform your business, you should undeniably integrate AI with IoT devices.
Recent Posts
December, 2021